About the Project
Graph-Massivizer
Graph-Massivizer researches and develops a high-performance, scalable, and sustainable platform for information processing and reasoning based on the massive graph representation of extreme data. It delivers a toolkit of five open-source software tools and FAIR graph datasets covering the sustainable lifecycle of processing extreme data as massive graphs. The tools focus on holistic usability (from extreme data ingestion and massive graph creation), automated intelligence (through analytics and reasoning), performance modelling, and environmental sustainability tradeoffs, supported by credible data-driven evidence across the computing continuum.
Consortium
Graph-Massivizer gathers a consortium of twelve partners from eight countries, covering four academic universities, two applied research centres, one HPC centre, two SMEs and two large enterprises. It leverages the world-leading roles of European researchers in graph processing and serverless computing and uses leadership-class European infrastructure in the computing continuum.
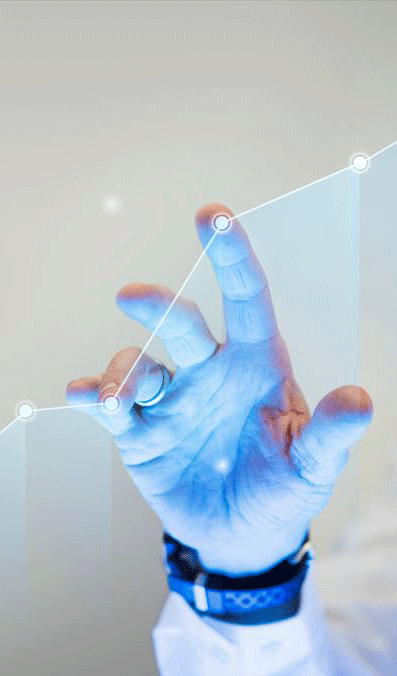
Graph-Massivizer Software Tools

The project delivers the Graph-Massivizer toolkit of five open-source software (OSS) tools and FAIR graph datasets covering the sustainable lifecycle of processing extreme data as MG.
The tools focus on holistic
1. Usability
2. Automated intelligence
3. Performance modelling
4. Environmental sustainability tradeoffs supported by credible data-driven evidence
5. Across HPC systems and computing continuum
Graph-Massivizer Software Tools
The project delivers the Graph-Massivizer toolkit of five open-source software (OSS) tools and FAIR graph datasets covering the sustainable lifecycle of processing extreme data as MG.
The tools focus on holistic
1. Usability
2. Automated intelligence
3. Performance modelling
4. Environmental sustainability tradeoffs supported by credible data-driven evidence
5. Across HPC systems and computing continuum
News
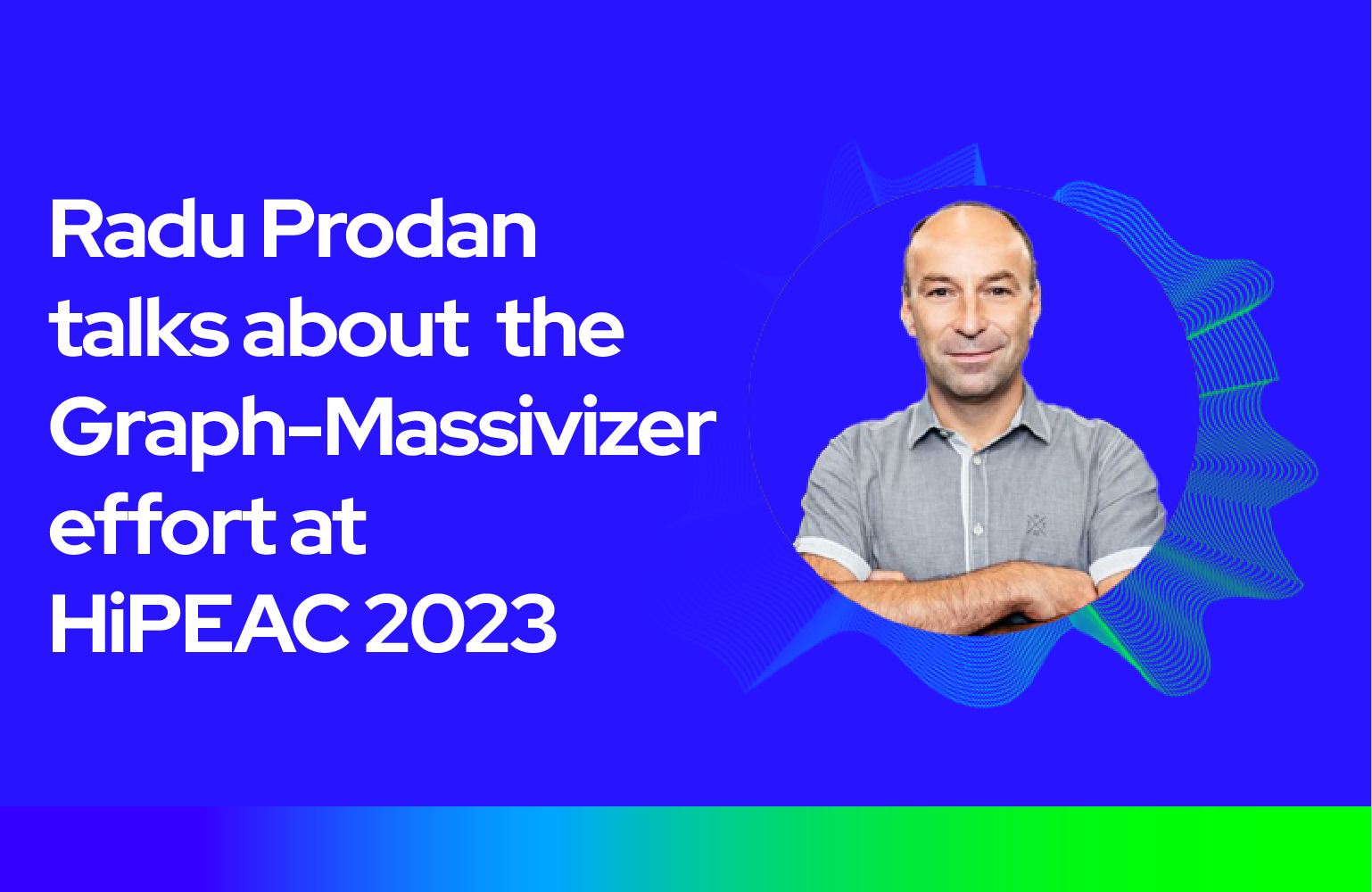
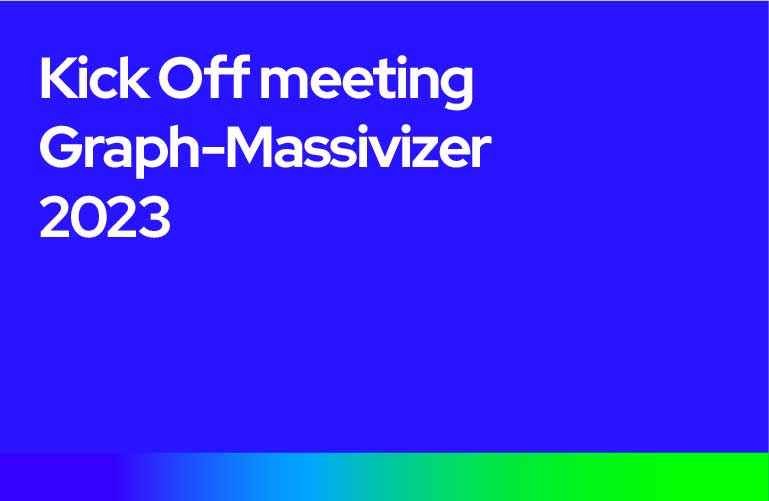
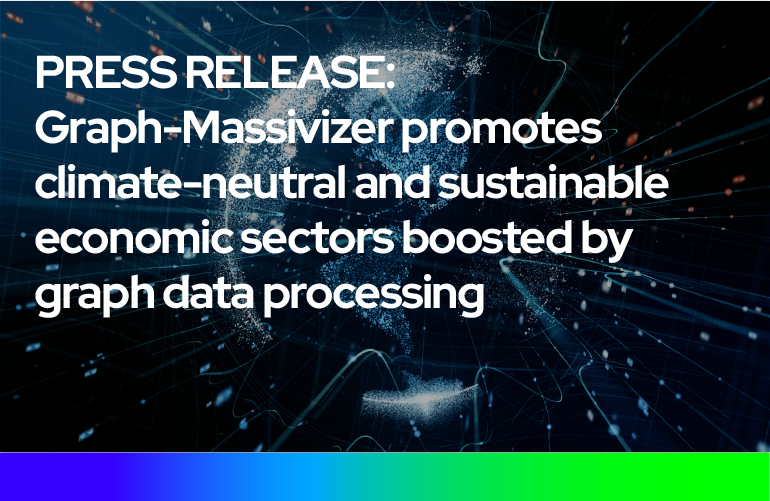
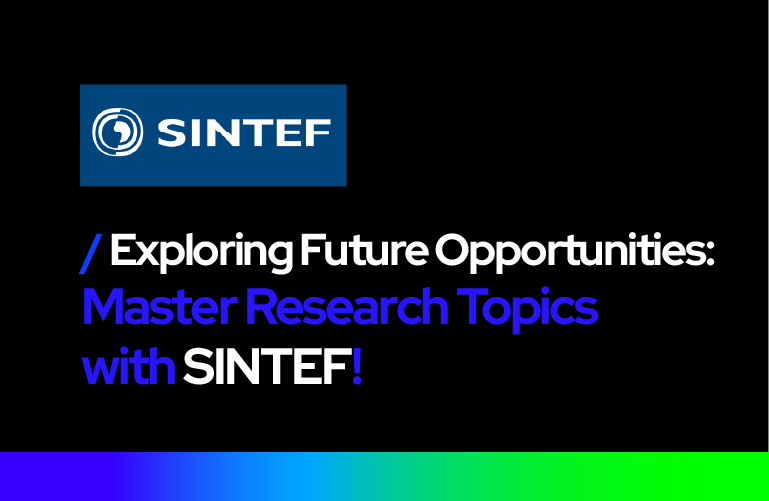
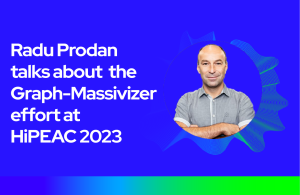
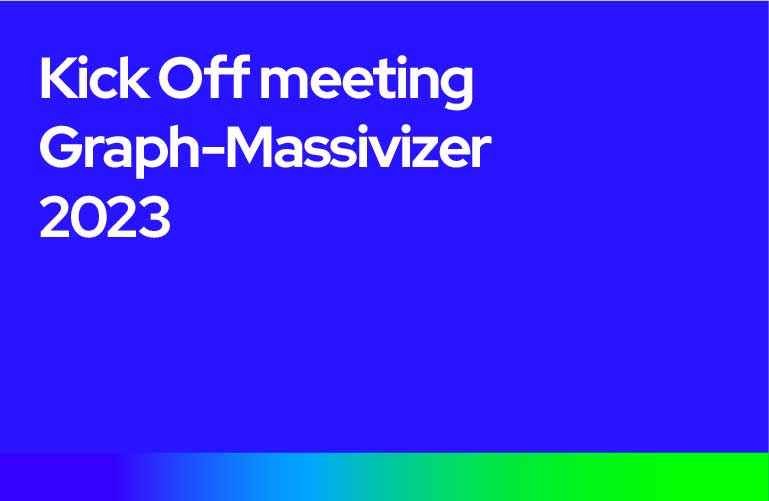
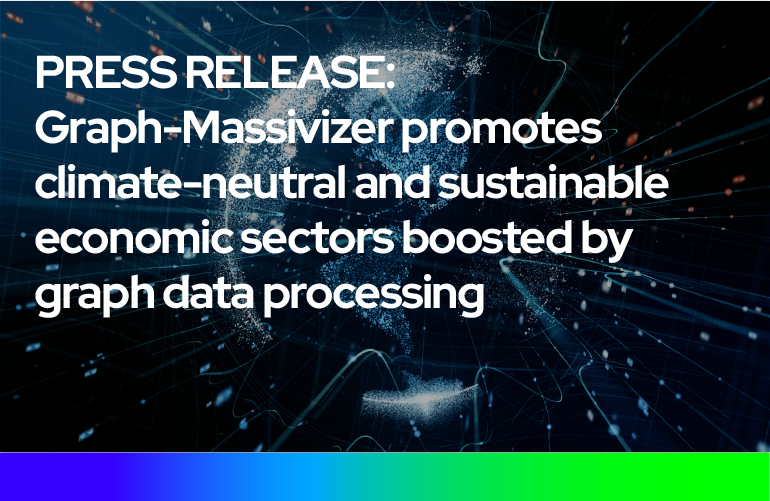
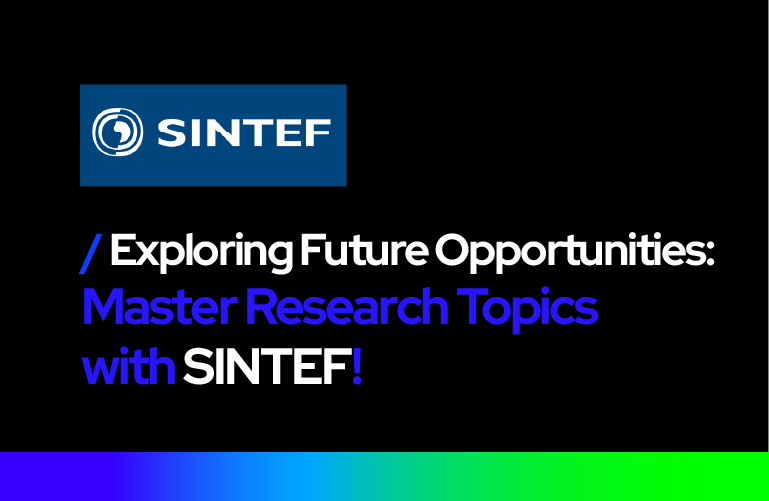
Blog

GraphMa: Graph Processing with Pipeline-Oriented Computation
In the realm of data science and analytics, the significance of graph processing cannot be overstated. The intricate web of relationships and connections that graphs represent are fundamental to understanding complex systems, from social networks to biological...

How we implemented scalable graph summarization
tl;dr k-bisimulation can be used to create a condensed version of a graph. This condensed version is a graph summary, keeping specific properties of the original k-bisimulation partitions the nodes of the graph in equivalence classes which we call blocks We create the...

Neurosymbolic quality monitoring for sustainable manufacturing
Graph Massivizer will be a big step towards harmonizing neural and symbolic AI methods, a revolutionary approach to quality estimation in welding processes, and a profound leap towards realizing a genuinely sustainable automotive industry. In the fast-paced world of...

Graph sampling algorithms and predicting their qualities and runtimes
What is graph sampling? With the growing size of graphs in the real world, reducing their complexities becomes increasingly essential and computationally demanding, and due to the unavailability of whole graphs for privacy or scalability reasons, gathering relevant...

Graph massivizer: much more than another Graph Database Platform
Graph databases are gaining traction in the data management landscape for their ability to handle complex relationships and networks. However, Graph Massivizer stands out as more than just another graph database platform. It offers unique features and capabilities...
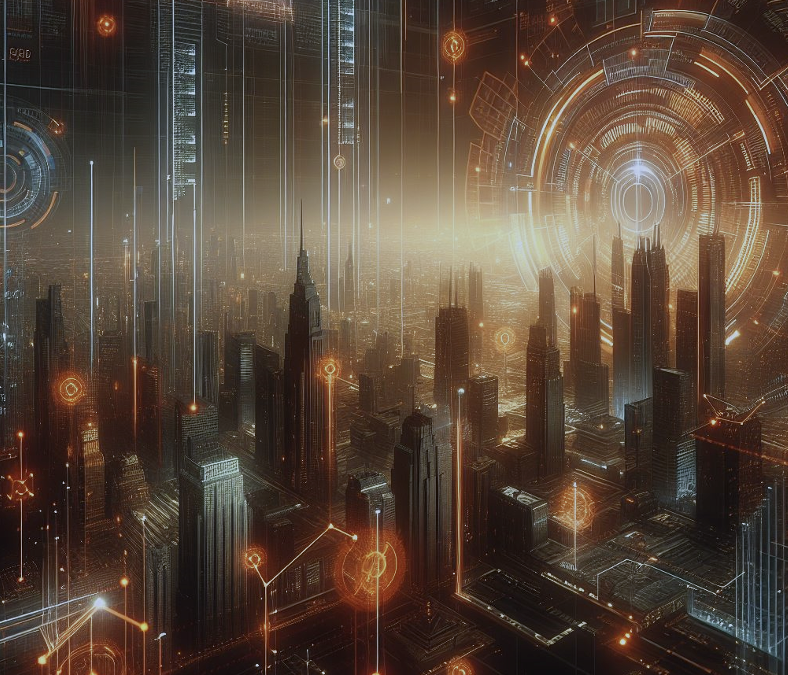
Synthetic Data Powered Investment and Trading
Author: Laurentiu Vasiliu Peracton Ltd. In the ever-evolving world of finance, Synthetic Data Driven Investment and Trading is emerging as hybrid approach, where financial algorithms are not only powered by traditional financial data (historic and live), but also by...
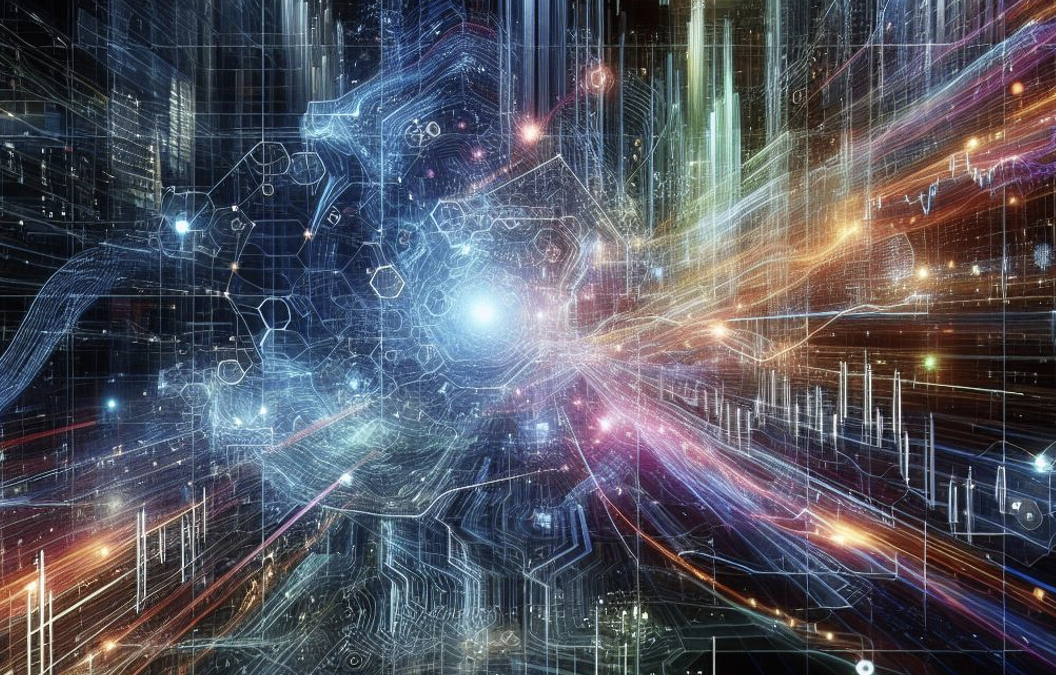
Trading in the Matrix
Author: Laurentiu Vasiliu Peracton Ltd. Imagine a scenario where extreme quantities of synthetic data are continuously generated and used to train multiple generations of AI enhanced financial algorithms. In this scenario, financial algorithms train on making...
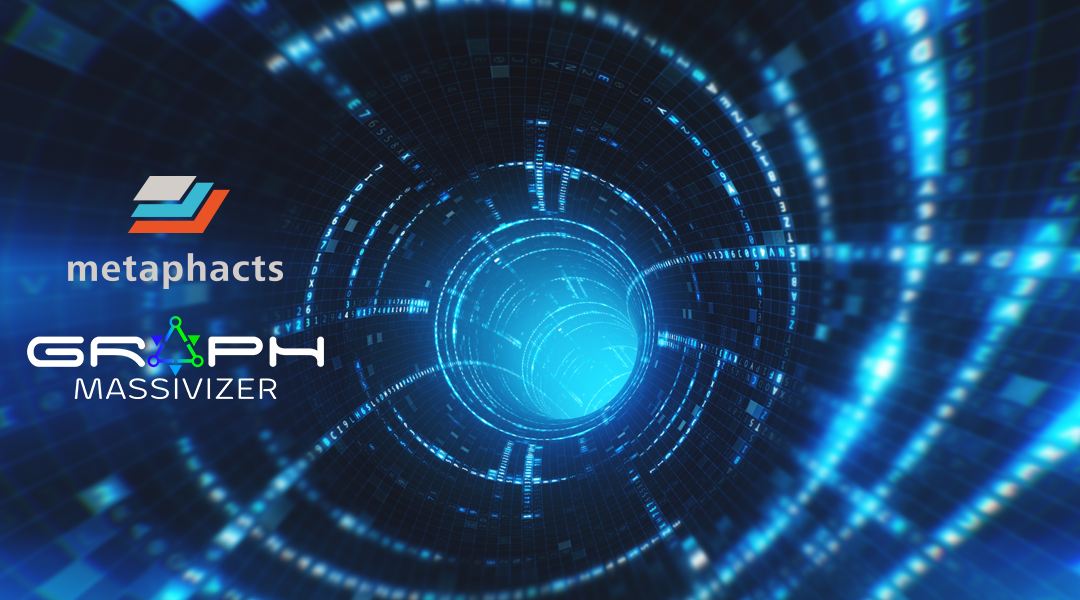
Building massive knowledge graphs using automated ETL pipelines
In this blog post, written by our colleagues Wolfgang Schell and Pauline Lencio at metaphacts , the Graph-Massivizer team explains how to build a massive knowledge graph from existing information or external sources in a repeatable and scalable manner. The post...
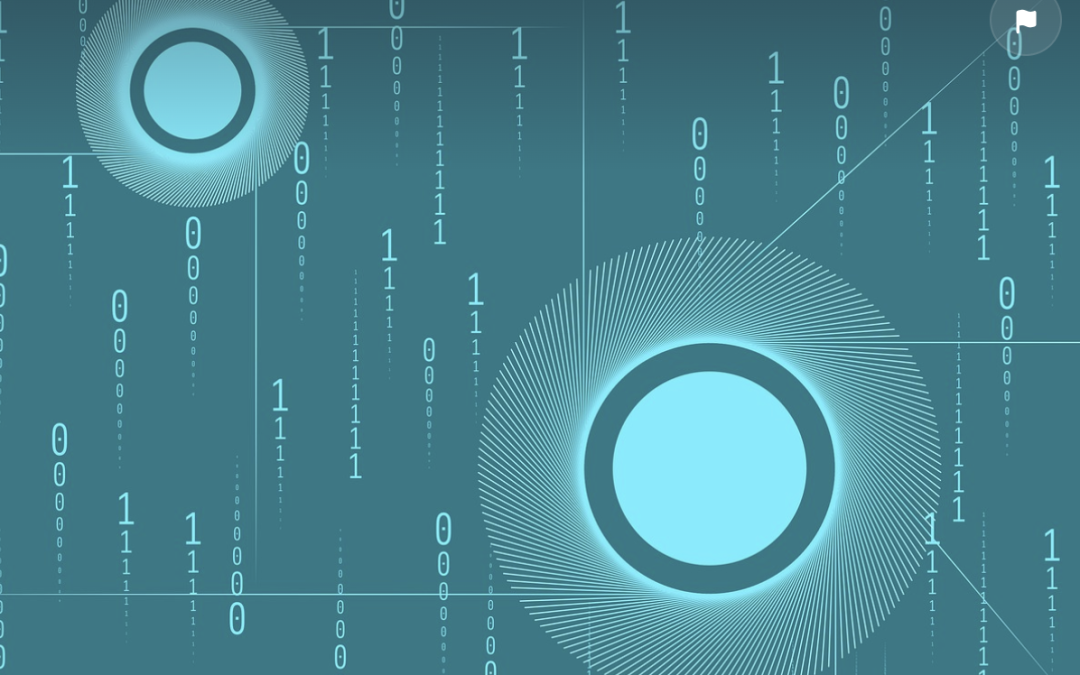
From Big Data to Green Data: Reducing the Environmental Impact of Data Science with Graph Massivizer
Data analysis and data processing are technologies that are increasingly prevalent in everyday life, with applications in research, industry, commerce, and public administration. However, they also have a significant environmental impact, both direct and indirect, due...
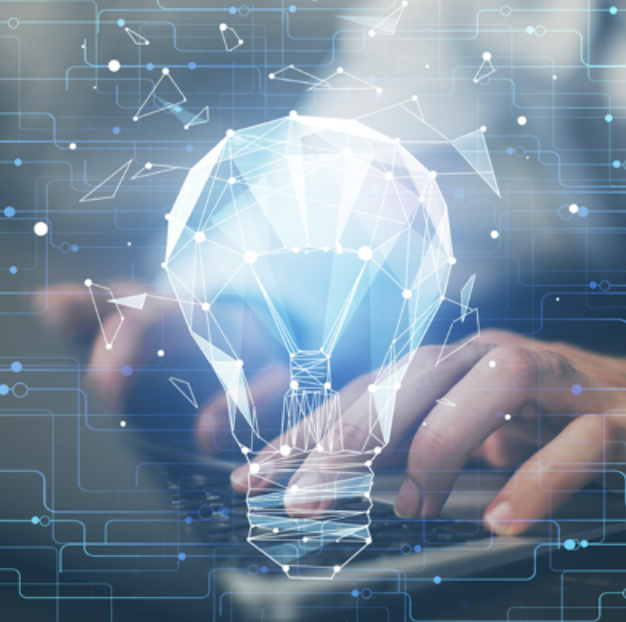
The importance of the semantic knowledge graph
This article is the first in a series of two where we discuss our perspective on what is considered a semantic knowledge graph, why it’s important, and share how they can drive your enterprise goals forward.
