Green AI for Sustainable Automotive Industry
The automotive value chain involves extreme data flows of heterogeneous, distributed, fast-growing, disconnected, or hardly compatible information. ML methods face new challenges and opportunities to holistically analyse the massive and unprecedented data integrated across these chains, to support decisions that fundamentally change automotive manufacturing processes towards a sustainable, circular, and climate-neutral automotive industry. Graph-Massivizer enables new graph-based encoding that captures several value-chain stages to predict their outcome better and detect anomalies. Better and quicker analysis prevents defect propagation and unnecessary waste, contributing to a sustainable, circular, and climate-neutral automotive industry. By combining graph-based ML methods with digital twins, Graph-Massivizer provides new insights and boosts the efficiency and scalability of the diagnosis beyond that of more expensive alternatives (e.g., excessive sensor deployment for continuous monitoring).
Objective
Predict “best” production configurations for a given BiW type and welding machines over simulated data with predictable manufacturing KPIs (BiW quality).
Result
The Green AI for Sustainable Automotive Industry Use Case in Detail
The automotive industry involves a complex network of data flows that are often incompatible and diverse. To address this issue, we plan to adopt the Graph-Massivizer toolkit, which uses Machine Learning (ML) methods to analyze this data holistically. This will pave the way for a sustainable, circular, and climate-neutral automotive industry.
Graph-Massivizer uses a unique graph-based encoding that captures multiple value-chain stages, improves prediction accuracy, and anomaly detection. This enhanced analysis will help to reduce defects and waste, promoting industry sustainability. By integrating Graph-Massivizer’s graph-based ML methods with digital twins, we aim to improve diagnostic efficiency and scalability, surpassing more expensive alternatives such as continuous sensor monitoring.
The primary objective of this Use Case is to develop a solution that can predict the best production configurations for a given material type and welding machines. We will use simulated data to achieve the desired quality levels.
The Use Case aims to improve the accuracy of predicting the produced spot diameter based on an input welding program during simulation. This will help in determining the quality of the welding result and predicting the parameters required to produce a specific welding quality.
The logic or workflow is as follows. Firstly, the sensor data, welding machines, welding programs, and quality specifications are onboarded. Then, these are mapped to the welding quality ontology. Next, an algorithm is chosen (such as a neural algorithm) and a graph embedding model is trained. Once the algorithm is selected, the necessary hardware resources for the algorithm and data are acquired. Finally, the algorithm is executed, and its performance is monitored.
We plan to use the GM platform to effectively manage the streams of data produced by the numerous sensors that monitor the welding process and the machines used. This data will be integrated with other sources in the graph, and symbolic and sub-symbolic reasoning will be applied on the graph. To facilitate the integration of the sensor data in the KG, the Graph Inceptor is crucial. The Scrutinizer will be utilized to mine the produced graph. We also intend to use the Optimizer and the Greenified to pick the best resources for the selected algorithm. This will help us avoid overusing resources and most importantly, reduce failures and repeated computations caused by insufficient resources.
Expected Outcomes of the Use Case
The expected results will be obtained by generating KG embedding of the constructed welding graph and using the embedding model to predict the quality of the welding spot. This will also help in determining the most suitable program for a given material and spot.
The results will be presented in the form of an interactive view of the developed ontology and a graph generated from synthesized data. Additionally, there will be a query interface available for stakeholders to test the system and obtain the desired results.
The plan for exploitation involves the extension of the project output to create an internal platform for industrial data that is similar to the welding scenario. The aim is to adapt and expand this output to more Bosch plants, thereby addressing the needs of the industrial floors. The stakeholders involved are multiple Bosch plants that are involved in welding procedures. The sensor data is expected to provide inferred information that can assist technicians, engineers, and managers in optimizing the production process.
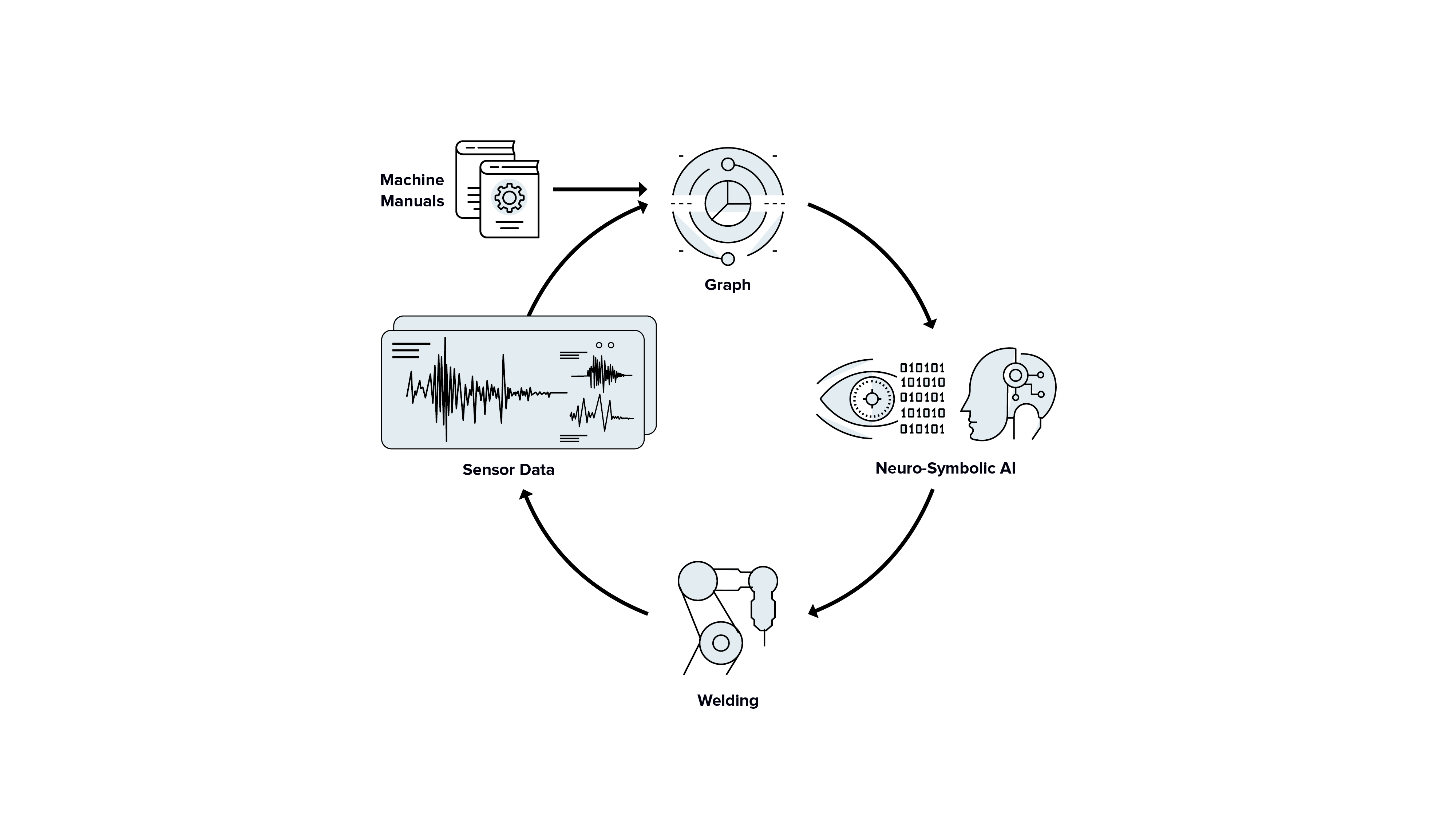
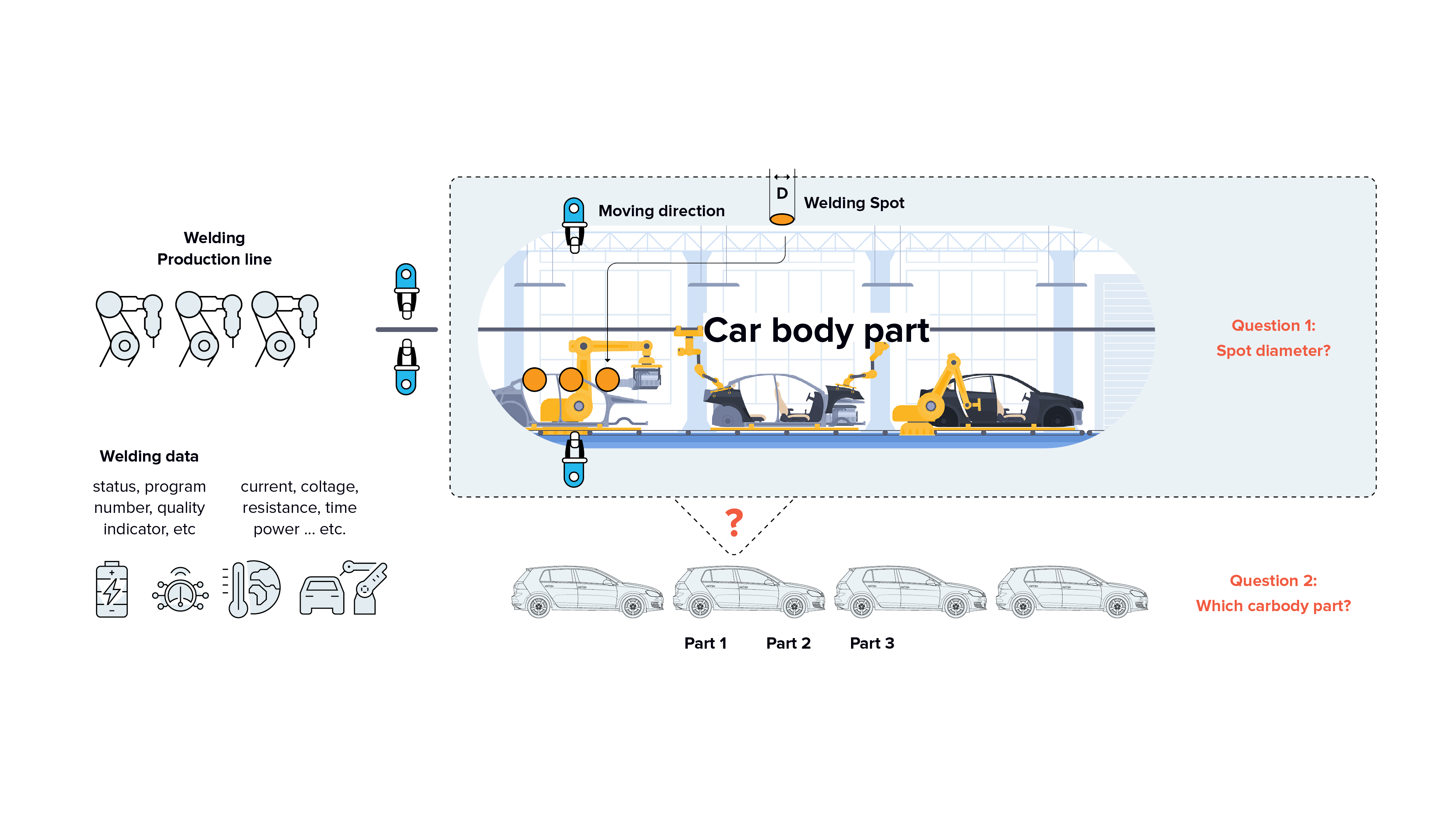
