Green and Sustainable Finance
Graph-Massivizer aims to remove the limitations of financial market data providers (limited volume, reduced accessibility, very high costs, limited historic relevance) by enabling fast semi-automated creation of realistic and affordable synthetic extreme financial data sets, unlimited in size and accessibility. Peracton Ltd. uses the financial multiverse for improved AI-enhanced green investment and trading simulations, free of critical biases such as prior knowledge, over-fitting, and indirect contamination due to historic financial data scarcity and limitations. There are two major objectives to be achieved:
Objective 1
Generate an energy-efficient synthetic financial data (multiverse), validated using standard (green) financial investment and trading algorithms against real historical financial data sets.
Result
Green Financial Data Multiverse sustainable extreme data archive.
Objective 2
Use Financial Data Multiverse for improved green AI-enhanced financial algorithms with reduced bias, risk, and higher performance.
Result
Improved green financial algorithm portfolio for better investment returns and lower risk.
The Green and Sustainable Finance Use Case in detail
One goal of the Graph-Massivizer project is to eliminate the constraints posed by financial market data providers, such as limited volume, restricted accessibility, high costs, and limited historical relevance of historic data. The project achieves this by facilitating the rapid semi-automated generation of realistic and cost-effective synthetic financial datasets in massive volumes (petabytes), with limitless scalability and accessibility.
What is synthetic data? Synthetic data is artificially generated data that mimics real-time and historical data in terms of essential characteristics, capturing and reflecting the statistical properties of real-world data. In the context of investment and trading, synthetic data is useful for simulating various market conditions and investment scenarios, providing a rich and diverse dataset for analysis.
It can be used for different main topics at the heart of investment and trading, such as:
Stress Testing Financial Algorithms
Stress testing financial algorithms means that algorithms are exposed to a multitude of extreme market conditions, sudden economic shifts, and unforeseen geopolitical events. Rigorous and in-depth stress testing helps identify potential weaknesses and vulnerabilities, enabling traders and investors to refine and fortify their algorithms pre-emptively.
Portfolio Optimization
Traders and fund managers can test various portfolio combinations and strategies under never-encountered-before market conditions, including green-type investments. This can lead to the creation of more robust and diversified portfolios that can withstand market volatility and deliver consistent returns.
Modeling Market Anomalies
Market anomalies, such as sudden price jumps or crashes, can significantly impact investment and trading performance. Synthetic data can help model these anomalies by simulating their occurrence and studying their impact on various investment strategies. This can enable traders, investors, and fund managers to devise strategies to mitigate the impact of these anomalies.
Risk Management
Furthermore, Risk Management is critical to any investment and trading strategy. Synthetic data can enhance risk management by comprehensively understanding various risk factors and their interplay under different market conditions. This can help traders and fund managers manage risk better and protect their investments.
Synthetic data for algorithmic trading testing and modeling represents a significant paradigm shift, particularly when extreme volumes are used for such testing. This approach unlocks many new possibilities, allowing the development of adaptable and more robust trading and investment algorithms.
Use case overview
The primary outcomes of this use case include a flexible Graph-Massivizer software platform capable of generating customized synthetic data batches for various types of securities, an extensive synthetic dataset (petabytes in size) ready for testing, encompassing USA stocks (S&P500) and commodities such as Wheat, Corn, and Soya. Free samples of the synthetic dataset will be provided.
The exploitation strategy for the proposed solution includes licensing, API access fees, white-labeling, partnerships, and integrations for the Graph-Massivizer platform. Data monetization is also anticipated for bespoke synthetic datasets.
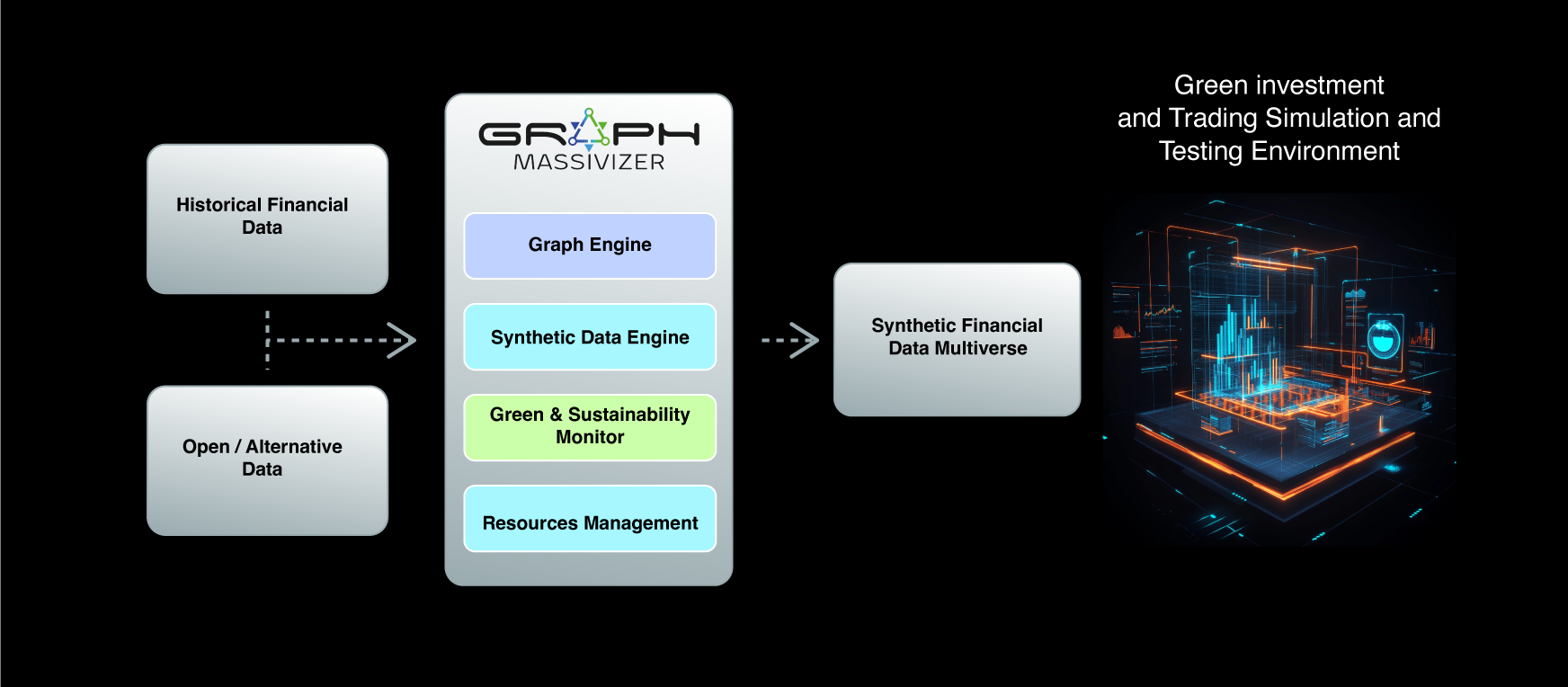
